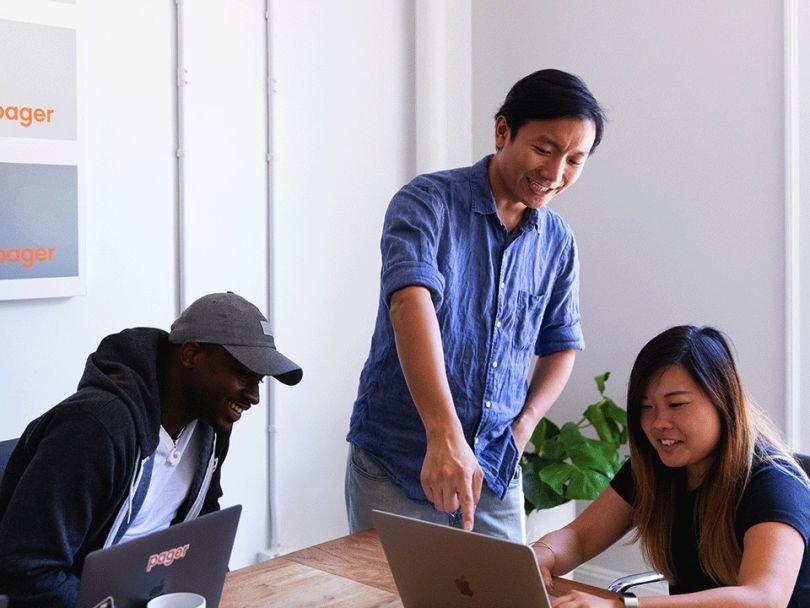
Plenty of applications promise to transform businesses, but not all predictive models actually cut costs, increase revenue or improve customer and employee experiences.
Successfully deploying — and monitoring — machine learning solutions requires businesses to experiment with processes and tools while building upon best practices from other tech teams in the industry. We spoke to professionals at two New York tech companies to learn more about their best practices for ML model deployment and maintenance.
“New model architectures are created almost daily, but often the purported gains of such approaches fail to outweigh the technical debt,” Machine Learning Research Engineer Alex Ruch said.
Ruch works at Graphika, which uses AI to map online social landscapes. He recommends that data teams do a cost-benefit analysis before deploying new models. Oftentimes, he said time is better spent investing energy into optimizing quality data to ensure both present and future models have the highest chance of working efficiently.
At Pager, another NYC tech company, user input plays a vital role in how models are productionized within design features of the company’s telehealth service platform. Data Engineer Jaime Ignacio Castro Ricardo said proof-of-concept feedback is integrated into models and users take notice.
“Users are usually happy when models are deployed since they were involved in feature planning, discussion and reviews,” Ricardo said.
The two ML experts dove into how their teams productionize machine learning models that work for their businesses, below.
What tools have you found to be most effective for productionizing Graphika’s ML models?
We use a variety of Python-based ML frameworks to examine how behavior and language unfold over the cybersocial landscapes of our network maps. Many of our deep learning models use PyTorch as a back end. For example, the Deep Graph Library provides a flexible way for us to develop semi-supervised classification models for nodes. And the DGL-KE package lets us scale our knowledge graphs to millions of nodes. Hugging Face’s Tokenizers and Transformers libraries also enabled us to produce and test language models for text classification and multi-label models of sentiment while avoiding huge amounts of boilerplate code.
These tools greatly enhanced our ability to quickly generate results given their easy integration with GPU processing. But we also use more traditional ML frameworks like scikit-learn, Gensim, and scattertext for in-house analyses.
Teams should carefully evaluate when the benefits of new models outweigh their costs.”
What are your best practices for deploying a machine learning model to production?
We use MLflow to package, track, register and serve machine learning projects. It’s helped us make improvements to ensure model integrity while letting us efficiently replicate runtime environments across servers. For example, MLflow automatically logs our automated hyperparameter tuning trials with Optuna. It also saves the best-performing model to our registry along with pertinent information on how and on what data it was trained.
Then, MLflow allows us to easily serve models accessible by API requests. Together, this training and deployment pipeline lets us know how each of our models were created. It helps us better trace the root cause of changes and issues over time as we acquire new data and update our model. We have greater accountability over our models and the results they generate.
What advice do you have for other data scientists looking to better productionize ML models?
Productionizing machine learning models is a complex decision-making process. New model architectures are created almost daily, but often the purported gains of such approaches fail to outweigh the technical debt. For example, a simple logistic regression model can often perform within an acceptable range compared to a deep neural network if data is high quality.
Teams should carefully evaluate when the benefits of new models outweigh their costs, and when they should update or upgrade modeling approaches. Perhaps effort would be better spent on improving data quality, which would not only help the present modeling pipeline but boost the performance of future models. This approach minimizes technical debt now and in the future, whereas changing models may only affect immediate performance gaps.
What tools have you found to be most effective for productionizing Pager’s ML models?
Like most of the industry, we use Python and Jupyter Notebooks for exploratory data analysis and model development. We recently switched from self-hosting Jupyter Notebooks to using Google Colab since much of our tech stack is already on the Google Cloud Platform. Colab offers an easy medium for collaboration between team members.
We deal primarily with chatbots, so our ML stack is geared toward natural language processing. We use scikit-learn, spaCy, and Rasa as the main ML and NLP libraries to build our models. There’s also an in-house framework we developed around them to streamline our experimentation and deployment process.
The engineering department integrated GitOps into our continuous integration and delivery pipelines. New versions of our models are Dockerized and deployed to a production Kubernetes cluster when we merge into master by Google Cloud Build.
User, client and clinical input gets used to design product features that leverage our models.”
What are your best practices for deploying a machine learning model to production?
We perform thorough unit testing with pytest, and exhaustive integration and user testing in multiple lower-level environments. User, client and clinical input gets used to design product features that leverage our models. Then we iterate on proof-of-concept feedback from users. We also quantify how new ML models affect efficiency and productivity to gauge their real-world effectiveness.
Because of these practices, our ML team rarely introduces bugs in production. Users are usually happy when models are deployed since they were involved in feature planning, discussion and reviews. Additional training and input helps users be more efficient when using the features in production.
What advice do you have for data scientists looking to better productionize ML models?
Always keep track of experiments with versioning; not just for trained models but also for the input data, hyperparameters and results. Such metadata proves useful when we develop new models for the same problem and reproduce old ones for comparison and benchmarking. We use an in-house framework for developing new models, but MLflow is a great open-source solution as well.